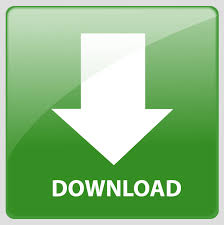
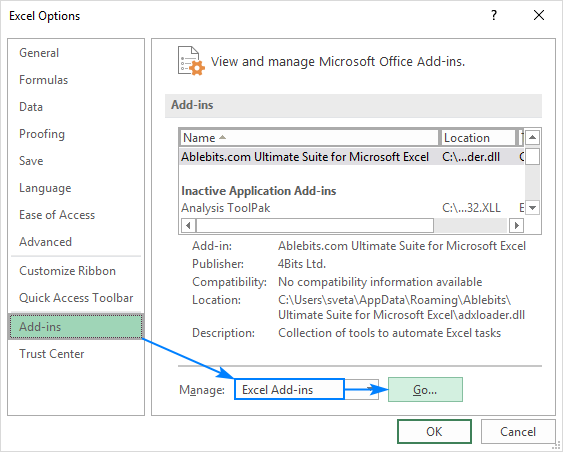
Arguably a better way of describing R squared is as a measure of 'explained variation'. This simple example illustrates that, although R squared is an important measure, a high value does not mean our model is correctly specified. This is obviously a consequence of the fact that the expectation of Y depends on exp(X), whereas the model we have used assumes it is a linear function of X. In particular we see that for both low and high values of X, the fitted values are too small. Overlaying the fitted line onto the observed data makes clear that the model we have used is not correctly specified, despite the fact that the R squared value is quite large. goodness of fit due to the addition of a certain term to the model bigger than the. However, if we again plot the observed data, and overlay it with the fitted line: Popular spreadsheet programs, such as Quattro Pro, Microsoft Excel. We might take this to mean that the model we have used, that the expectation of Y is linear in X, is reasonable. Also, we see that we obtain an R squared value of 0.46, indicating again that X (included linearly) explains quite a large amount of the variation in Y.
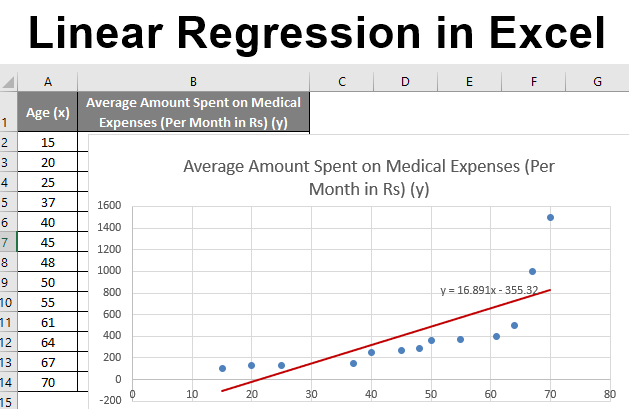
Unlike in the first case, the parameter estimates we obtain (1.65, 1.54) are not unbiased estimates of the parameters in the 'true' data generating mechanism, in which the expectation of Y is a linear function of exp(X). Residual standard error: 1.651 on 998 degrees of freedom
Excel linear regression determine quality of fit plus#
We then generate the outcome Y equal to X plus a random error, again with a standard normal distribution: n |t|) To do this, we randomly generate X values from a standard normal distribution (mean zero, variance one). This is easiest to illustrate with a simple example.įirst we will simulate some data using R.
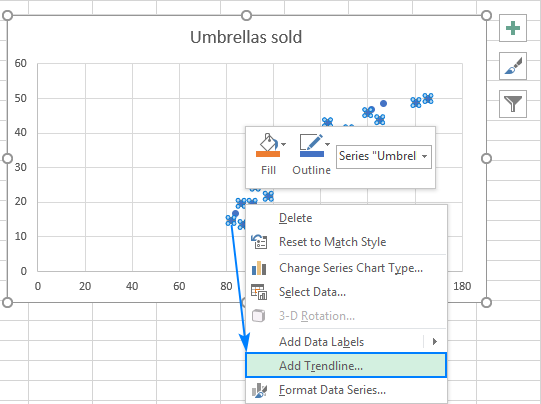
In particular, a high value of R squared does not necessarily mean that our model is correctly specified. That is, it does not tell us whether we have correctly specified how the expectation of the outcome Y depends on the covariate(s). This of course seems very reasonable, since R squared measures how close the observed Y values are to the predicted (fitted) values from the model.Īn important point to remember, however, is that R squared does not give us information about whether our model is correctly specified. R squared, the proportion of variation in the outcome Y, explained by the covariates X, is commonly described as a measure of goodness of fit. I’ve been teaching a modelling course recently, and have been reading and thinking about the notion of goodness of fit.
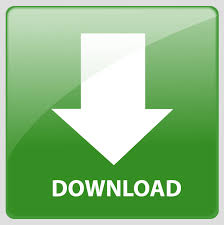